This is an easy-to-understand implementation of diffusion models within 100 lines of code. Different from other implementations, this code doesn't use the lower-bound formulation for sampling and strictly follows Algorithm 1 from the DDPM paper, which makes it extremely short and easy to follow. There are two implementations: conditional
and unconditional
. Furthermore, the conditional code also implements Classifier-Free-Guidance (CFG) and Exponential-Moving-Average (EMA). Below you can find two explanation videos for the theory behind diffusion models and the implementation.
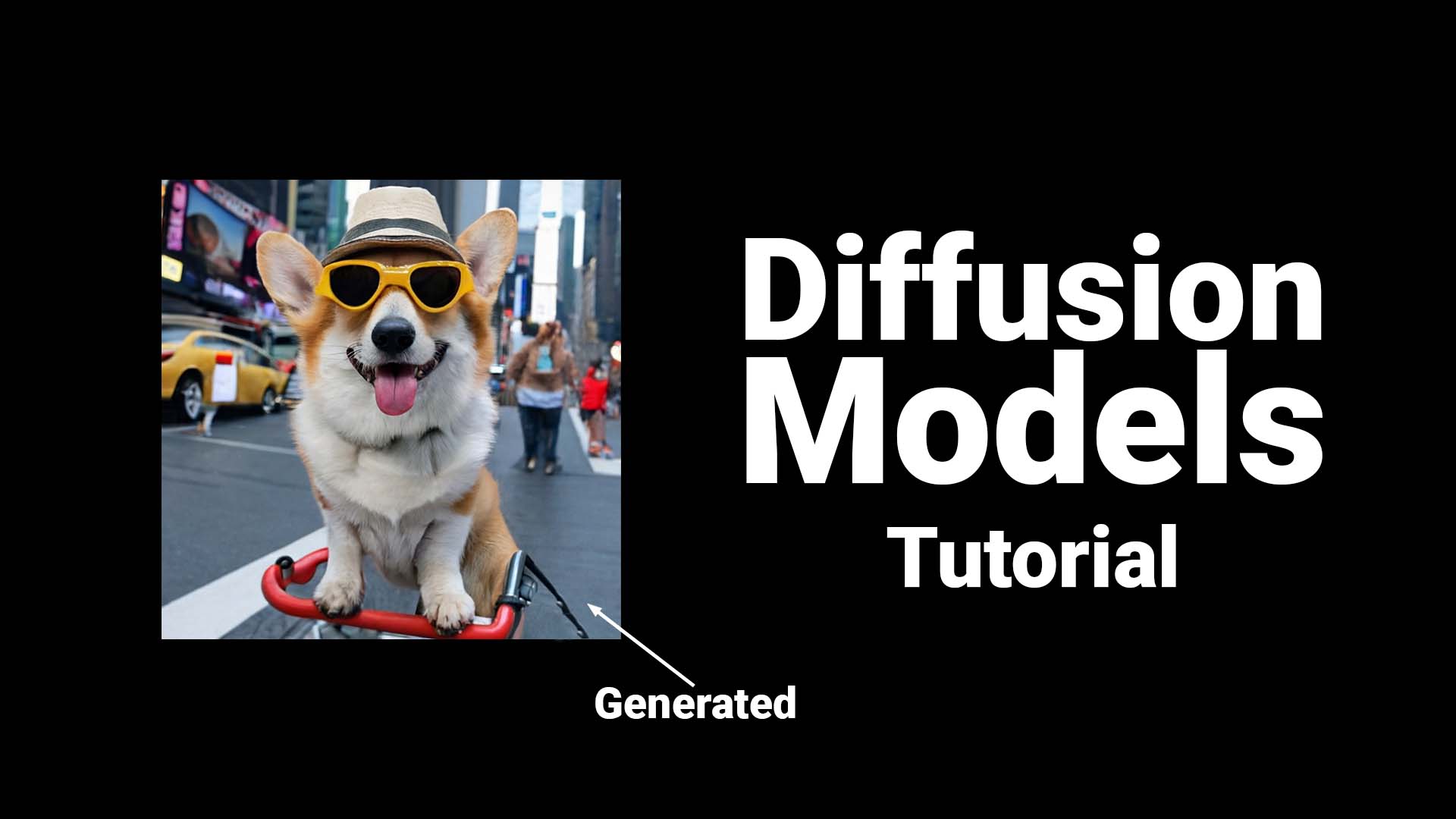
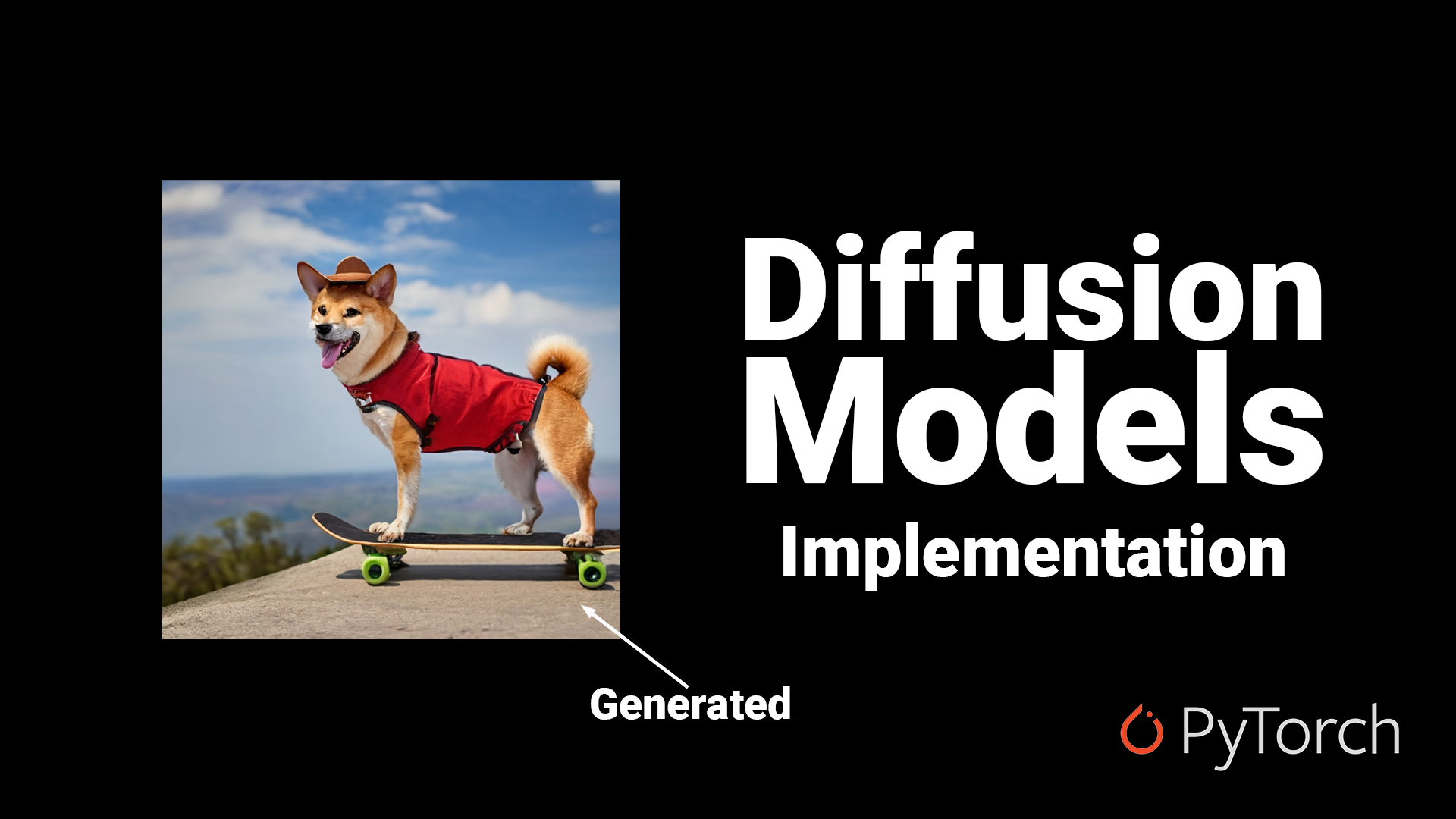
- (optional) Configure Hyperparameters in
ddpm.py
- Set path to dataset in
ddpm.py
python ddpm.py
- (optional) Configure Hyperparameters in
ddpm_conditional.py
- Set path to dataset in
ddpm_conditional.py
python ddpm_conditional.py
The following examples show how to sample images using the models trained in the video on the Landscape Dataset. You can download the checkpoints for the models here.
device = "cuda"
model = UNet().to(device)
ckpt = torch.load("unconditional_ckpt.pt")
model.load_state_dict(ckpt)
diffusion = Diffusion(img_size=64, device=device)
x = diffusion.sample(model, n=16)
plot_images(x)
This model was trained on CIFAR-10 64x64 with 10 classes airplane:0, auto:1, bird:2, cat:3, deer:4, dog:5, frog:6, horse:7, ship:8, truck:9
n = 10
device = "cuda"
model = UNet_conditional(num_classes=10).to(device)
ckpt = torch.load("conditional_ema_ckpt.pt")
model.load_state_dict(ckpt)
diffusion = Diffusion(img_size=64, device=device)
y = torch.Tensor([6] * n).long().to(device)
x = diffusion.sample(model, n, y, cfg_scale=3)
plot_images(x)
A more advanced version of this code can be found here by @tcapelle. It introduces better logging, faster & more efficient training and other nice features and is also being followed by a nice write-up.