-
Notifications
You must be signed in to change notification settings - Fork 668
Commit
This commit does not belong to any branch on this repository, and may belong to a fork outside of the repository.
[docs] Adds segment anything document (#3451)
- Loading branch information
Showing
3 changed files
with
293 additions
and
0 deletions.
There are no files selected for viewing
This file contains bidirectional Unicode text that may be interpreted or compiled differently than what appears below. To review, open the file in an editor that reveals hidden Unicode characters.
Learn more about bidirectional Unicode characters
This file contains bidirectional Unicode text that may be interpreted or compiled differently than what appears below. To review, open the file in an editor that reveals hidden Unicode characters.
Learn more about bidirectional Unicode characters
Original file line number | Diff line number | Diff line change |
---|---|---|
@@ -0,0 +1,173 @@ | ||
# Segment anything 2 example | ||
|
||
[Mask generation](https://huggingface.co/tasks/mask-generation) is the task of generating masks that | ||
identify a specific object or region of interest in a given image. | ||
|
||
In this example, you learn how to implement inference code with a [ModelZoo model](../../docs/model-zoo.md) to | ||
generate mask of a selected object in an image. | ||
|
||
The source code can be found | ||
at [SegmentAnything2.java](https://github.com/deepjavalibrary/djl/blob/master/examples/src/main/java/ai/djl/examples/inference/cv/SegmentAnything2.java). | ||
|
||
## Setup guide | ||
|
||
To configure your development environment, follow [setup](../../docs/development/setup.md). | ||
|
||
## Run segment anything 2 example | ||
|
||
### Input image file | ||
|
||
You can find the image used in this example: | ||
|
||
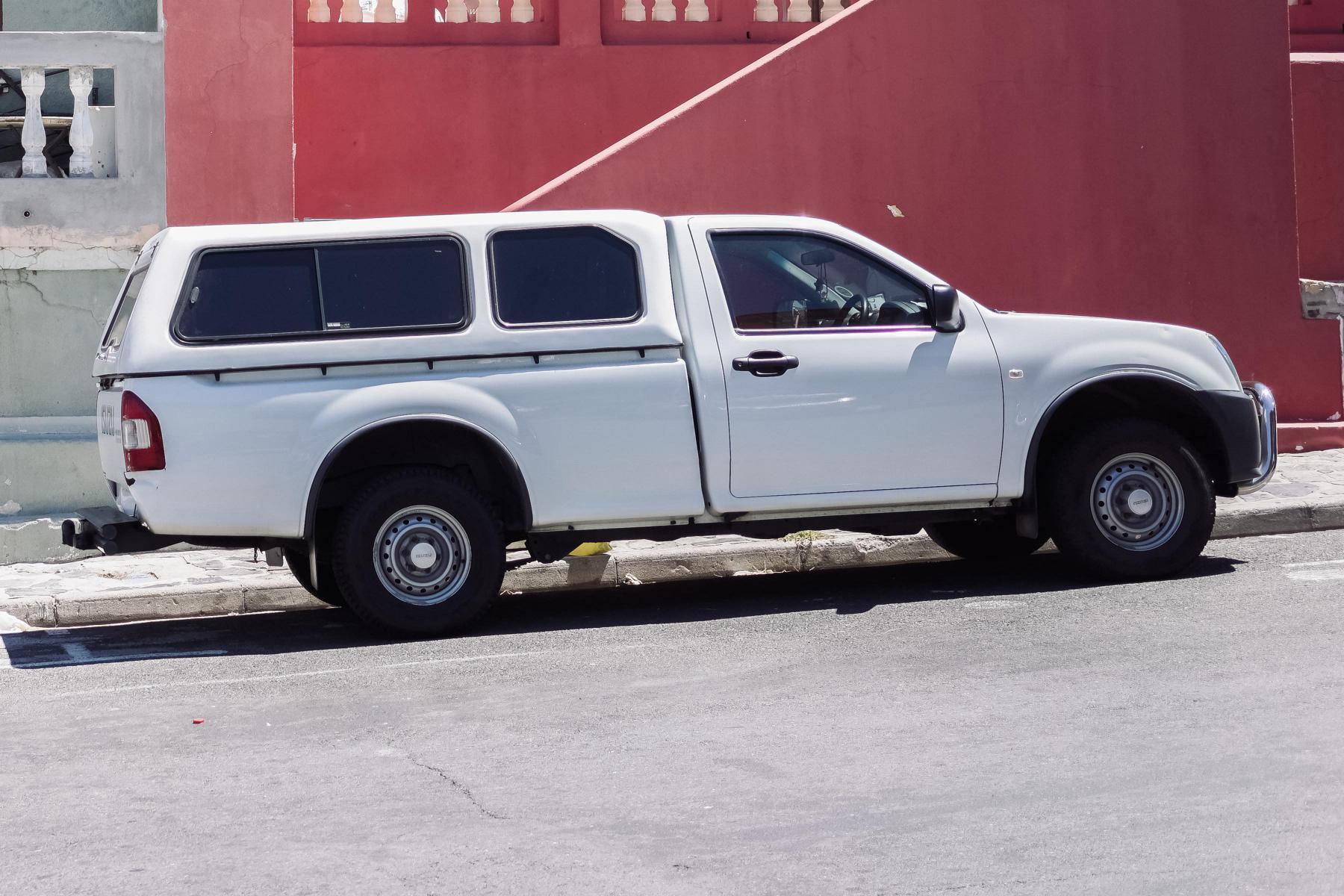 | ||
|
||
### Build the project and run | ||
|
||
Use the following command to run the project: | ||
|
||
```sh | ||
cd examples | ||
./gradlew run -Dmain=ai.djl.examples.inference.cv.SegmentAnything2 | ||
``` | ||
|
||
Your output should look like the following: | ||
|
||
```text | ||
[INFO ] - Number of inter-op threads is 12 | ||
[INFO ] - Number of intra-op threads is 6 | ||
[INFO ] - Segmentation result image has been saved in: build/output/sam2.png | ||
[INFO ] - [ | ||
{"class": "", "probability": 0.92789, "bounds": {"x"=0.000, "y"=0.000, "width"=1800.000, "height"=1200.000}} | ||
] | ||
``` | ||
|
||
An output image with bounding box will be saved as build/output/sam2.png: | ||
|
||
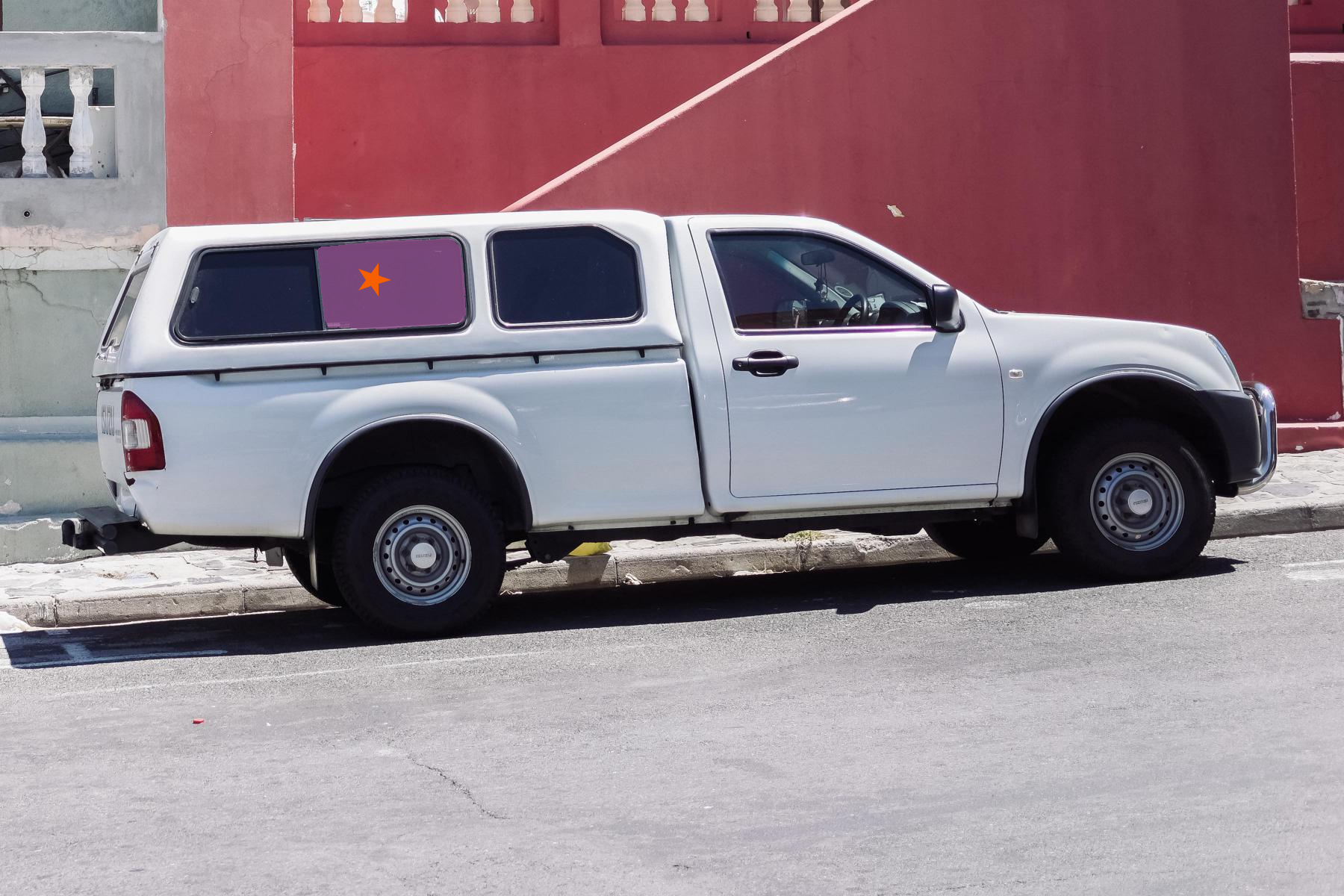 | ||
|
||
## Reference - how to import pytorch model: | ||
|
||
The original model can be found: | ||
|
||
- [sam2-hiera-large](https://huggingface.co/facebook/sam2-hiera-large) | ||
- [sam2-hiera-tiny](https://huggingface.co/facebook/sam2-hiera-tiny) | ||
|
||
The model zoo model was traced with `sam2==0.4.1` and `transformers==4.43.4` | ||
|
||
### install dependencies | ||
|
||
```bash | ||
pip install sam2 transformers | ||
``` | ||
|
||
### trace the model | ||
|
||
```python | ||
import sys | ||
from typing import Tuple | ||
|
||
import torch | ||
from sam2.modeling.sam2_base import SAM2Base | ||
from sam2.sam2_image_predictor import SAM2ImagePredictor | ||
from torch import nn | ||
|
||
|
||
class Sam2Wrapper(nn.Module): | ||
|
||
def __init__( | ||
self, | ||
sam_model: SAM2Base, | ||
) -> None: | ||
super().__init__() | ||
self.model = sam_model | ||
|
||
# Spatial dim for backbone feature maps | ||
self._bb_feat_sizes = [ | ||
(256, 256), | ||
(128, 128), | ||
(64, 64), | ||
] | ||
|
||
def extract_features( | ||
self, | ||
input_image: torch.Tensor, | ||
) -> (torch.Tensor, torch.Tensor, torch.Tensor): | ||
backbone_out = self.model.forward_image(input_image) | ||
_, vision_feats, _, _ = self.model._prepare_backbone_features( | ||
backbone_out) | ||
# Add no_mem_embed, which is added to the lowest rest feat. map during training on videos | ||
if self.model.directly_add_no_mem_embed: | ||
vision_feats[-1] = vision_feats[-1] + self.model.no_mem_embed | ||
|
||
feats = [ | ||
feat.permute(1, 2, | ||
0).view(1, -1, *feat_size) for feat, feat_size in zip( | ||
vision_feats[::-1], self._bb_feat_sizes[::-1]) | ||
][::-1] | ||
|
||
return feats[-1], feats[0], feats[1] | ||
|
||
def forward( | ||
self, | ||
input_image: torch.Tensor, | ||
point_coords: torch.Tensor, | ||
point_labels: torch.Tensor, | ||
) -> Tuple[torch.Tensor, torch.Tensor]: | ||
image_embed, feature_1, feature_2 = self.extract_features(input_image) | ||
return self.predict(point_coords, point_labels, image_embed, feature_1, | ||
feature_2) | ||
|
||
def predict( | ||
self, | ||
point_coords: torch.Tensor, | ||
point_labels: torch.Tensor, | ||
image_embed: torch.Tensor, | ||
feats_1: torch.Tensor, | ||
feats_2: torch.Tensor, | ||
) -> Tuple[torch.Tensor, torch.Tensor]: | ||
concat_points = (point_coords, point_labels) | ||
|
||
sparse_embeddings, dense_embeddings = self.model.sam_prompt_encoder( | ||
points=concat_points, | ||
boxes=None, | ||
masks=None, | ||
) | ||
|
||
low_res_masks, iou_predictions, _, _ = self.model.sam_mask_decoder( | ||
image_embeddings=image_embed[0].unsqueeze(0), | ||
image_pe=self.model.sam_prompt_encoder.get_dense_pe(), | ||
sparse_prompt_embeddings=sparse_embeddings, | ||
dense_prompt_embeddings=dense_embeddings, | ||
multimask_output=True, | ||
repeat_image=False, | ||
high_res_features=[feats_1, feats_2], | ||
) | ||
return low_res_masks, iou_predictions | ||
|
||
|
||
def trace_model(model_id: str): | ||
if torch.cuda.is_available(): | ||
device = torch.device("cuda") | ||
else: | ||
device = torch.device("cpu") | ||
|
||
predictor = SAM2ImagePredictor.from_pretrained(model_id, device=device) | ||
model = Sam2Wrapper(predictor.model) | ||
|
||
input_image = torch.ones(1, 3, 1024, 1024).to(device) | ||
input_point = torch.ones(1, 1, 2).to(device) | ||
input_labels = torch.ones(1, 1, dtype=torch.int32, device=device) | ||
|
||
converted = torch.jit.trace_module( | ||
model, { | ||
"extract_features": input_image, | ||
"forward": (input_image, input_point, input_labels) | ||
}) | ||
torch.jit.save(converted, f"{model_id[9:]}.pt") | ||
|
||
|
||
if __name__ == '__main__': | ||
hf_model_id = sys.argv[1] if len( | ||
sys.argv) > 1 else "facebook/sam2-hiera-tiny" | ||
trace_model(hf_model_id) | ||
``` |
This file contains bidirectional Unicode text that may be interpreted or compiled differently than what appears below. To review, open the file in an editor that reveals hidden Unicode characters.
Learn more about bidirectional Unicode characters
Original file line number | Diff line number | Diff line change |
---|---|---|
@@ -0,0 +1,119 @@ | ||
#!/usr/bin/env python | ||
# | ||
# Copyright 2024 Amazon.com, Inc. or its affiliates. All Rights Reserved. | ||
# | ||
# Licensed under the Apache License, Version 2.0 (the "License"). You may not use this file | ||
# except in compliance with the License. A copy of the License is located at | ||
# | ||
# http://aws.amazon.com/apache2.0/ | ||
# | ||
# or in the "LICENSE.txt" file accompanying this file. This file is distributed on an "AS IS" | ||
# BASIS, WITHOUT WARRANTIES OR CONDITIONS OF ANY KIND, express or implied. See the License for | ||
# the specific language governing permissions and limitations under the License. | ||
import sys | ||
from typing import Tuple | ||
|
||
import torch | ||
from sam2.modeling.sam2_base import SAM2Base | ||
from sam2.sam2_image_predictor import SAM2ImagePredictor | ||
from torch import nn | ||
|
||
|
||
class Sam2Wrapper(nn.Module): | ||
|
||
def __init__( | ||
self, | ||
sam_model: SAM2Base, | ||
) -> None: | ||
super().__init__() | ||
self.model = sam_model | ||
|
||
# Spatial dim for backbone feature maps | ||
self._bb_feat_sizes = [ | ||
(256, 256), | ||
(128, 128), | ||
(64, 64), | ||
] | ||
|
||
def extract_features( | ||
self, | ||
input_image: torch.Tensor, | ||
) -> (torch.Tensor, torch.Tensor, torch.Tensor): | ||
backbone_out = self.model.forward_image(input_image) | ||
_, vision_feats, _, _ = self.model._prepare_backbone_features( | ||
backbone_out) | ||
# Add no_mem_embed, which is added to the lowest rest feat. map during training on videos | ||
if self.model.directly_add_no_mem_embed: | ||
vision_feats[-1] = vision_feats[-1] + self.model.no_mem_embed | ||
|
||
feats = [ | ||
feat.permute(1, 2, | ||
0).view(1, -1, *feat_size) for feat, feat_size in zip( | ||
vision_feats[::-1], self._bb_feat_sizes[::-1]) | ||
][::-1] | ||
|
||
return feats[-1], feats[0], feats[1] | ||
|
||
def forward( | ||
self, | ||
input_image: torch.Tensor, | ||
point_coords: torch.Tensor, | ||
point_labels: torch.Tensor, | ||
) -> Tuple[torch.Tensor, torch.Tensor]: | ||
image_embed, feature_1, feature_2 = self.extract_features(input_image) | ||
return self.predict(point_coords, point_labels, image_embed, feature_1, | ||
feature_2) | ||
|
||
def predict( | ||
self, | ||
point_coords: torch.Tensor, | ||
point_labels: torch.Tensor, | ||
image_embed: torch.Tensor, | ||
feats_1: torch.Tensor, | ||
feats_2: torch.Tensor, | ||
) -> Tuple[torch.Tensor, torch.Tensor]: | ||
concat_points = (point_coords, point_labels) | ||
|
||
sparse_embeddings, dense_embeddings = self.model.sam_prompt_encoder( | ||
points=concat_points, | ||
boxes=None, | ||
masks=None, | ||
) | ||
|
||
low_res_masks, iou_predictions, _, _ = self.model.sam_mask_decoder( | ||
image_embeddings=image_embed[0].unsqueeze(0), | ||
image_pe=self.model.sam_prompt_encoder.get_dense_pe(), | ||
sparse_prompt_embeddings=sparse_embeddings, | ||
dense_prompt_embeddings=dense_embeddings, | ||
multimask_output=True, | ||
repeat_image=False, | ||
high_res_features=[feats_1, feats_2], | ||
) | ||
return low_res_masks, iou_predictions | ||
|
||
|
||
def trace_model(model_id: str): | ||
if torch.cuda.is_available(): | ||
device = torch.device("cuda") | ||
else: | ||
device = torch.device("cpu") | ||
|
||
predictor = SAM2ImagePredictor.from_pretrained(model_id, device=device) | ||
model = Sam2Wrapper(predictor.model) | ||
|
||
input_image = torch.ones(1, 3, 1024, 1024).to(device) | ||
input_point = torch.ones(1, 1, 2).to(device) | ||
input_labels = torch.ones(1, 1, dtype=torch.int32, device=device) | ||
|
||
converted = torch.jit.trace_module( | ||
model, { | ||
"extract_features": input_image, | ||
"forward": (input_image, input_point, input_labels) | ||
}) | ||
torch.jit.save(converted, f"{model_id[9:]}.pt") | ||
|
||
|
||
if __name__ == '__main__': | ||
hf_model_id = sys.argv[1] if len( | ||
sys.argv) > 1 else "facebook/sam2-hiera-tiny" | ||
trace_model(hf_model_id) |